
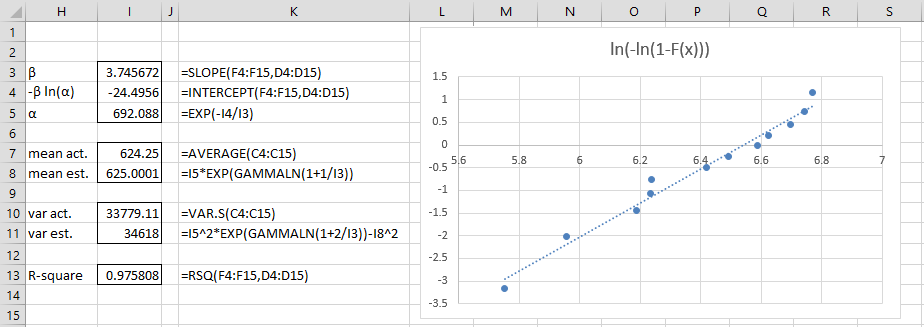
which means that the model is able to capture and learn from the non-linearity of the dataset. More than 98%+ Fitted values agree with the actual values. R-squared value has been improved and also In the above plots we can see the Actual vs Fitted values for Before and After assumption validations.
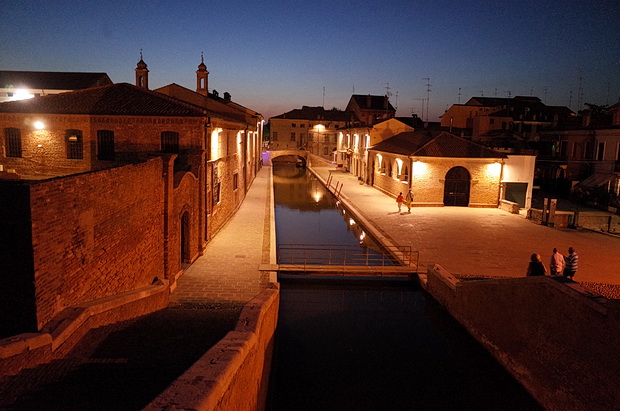
Also, you can use weighted least square method to tackle heteroskedasticity. We could do a non linear transformation of the dependent variable such as log(Y) or √Y. Residuals are nothing but the difference between actual and fitted values How to fix? If the plot shows a funnel shape pattern, then we say that Heteroskedasticity is present. Residual vs Fitted values plot can tell if Heteroskedasticity is present or not.
